Use Cases
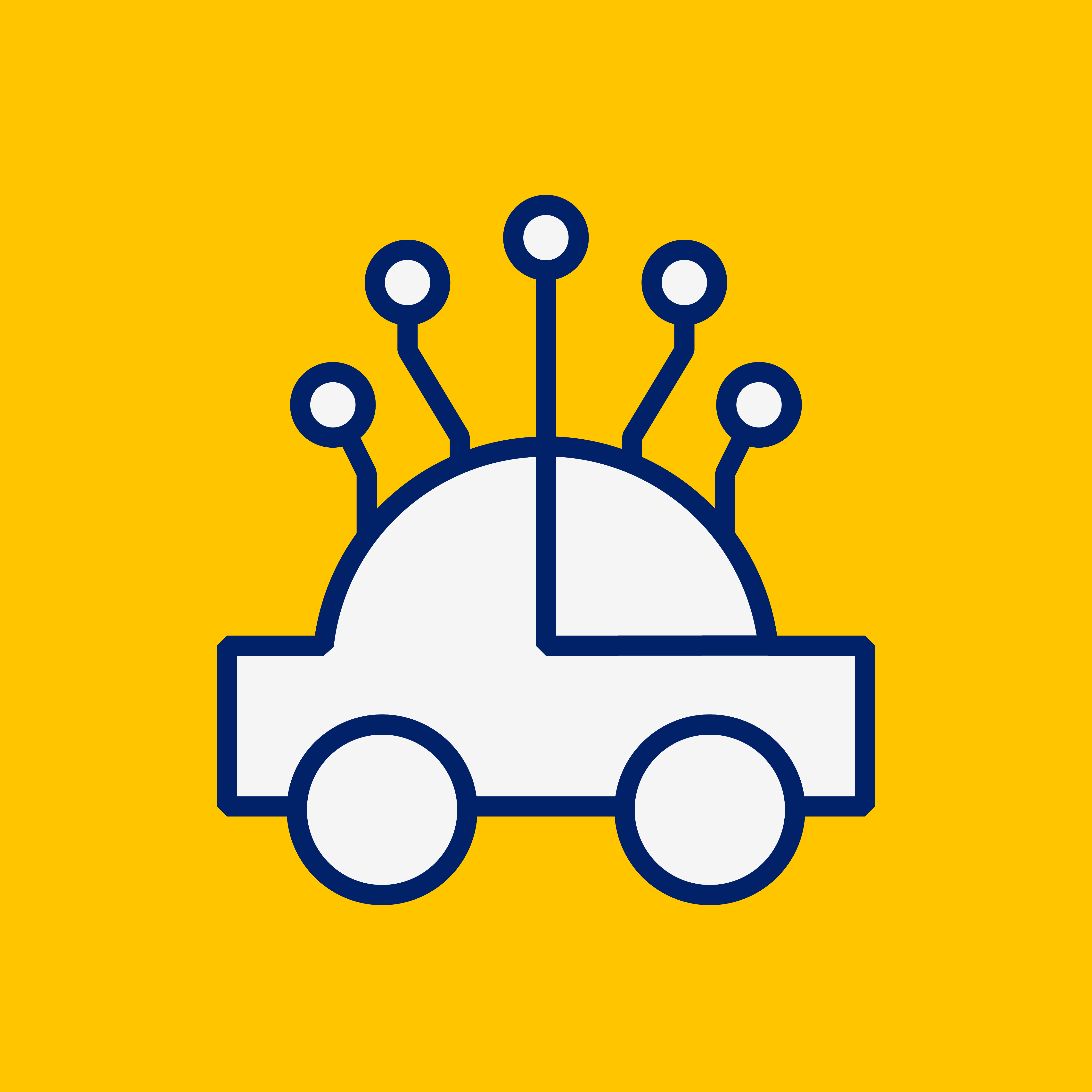
ELSA will leverage its broad industrial participation to develop six ambitious use-cases. The use-cases are central to our methodology and cover a wide spectrum of sectors where real-life impact of safe and secure AI is expected. Use cases will be used to articulate the research activities of the consortium, and initiate collaborations with external stakeholders. They will provide a yardstick to measure progress and drive research towards overcoming major obstacles in safe and secure AI. We have selected use cases that can be easily related to all grand challenges defined in this project, but we have decided to prioritise specific grand challenges with each use case (dark blue dots in the figure). This realistic approach ensures that all grand challenges are explored through at least two real-life use cases, while it leaves open the possibility to connect grand challenges with more use cases as the project advances. Each use case has two co-owners, aligning visions from academia and industry. In the following, we summarize the relevance of the six use cases to safe and secure AI and elaborate on the specific focus we will put on each one linking them to the identified grand challenges.
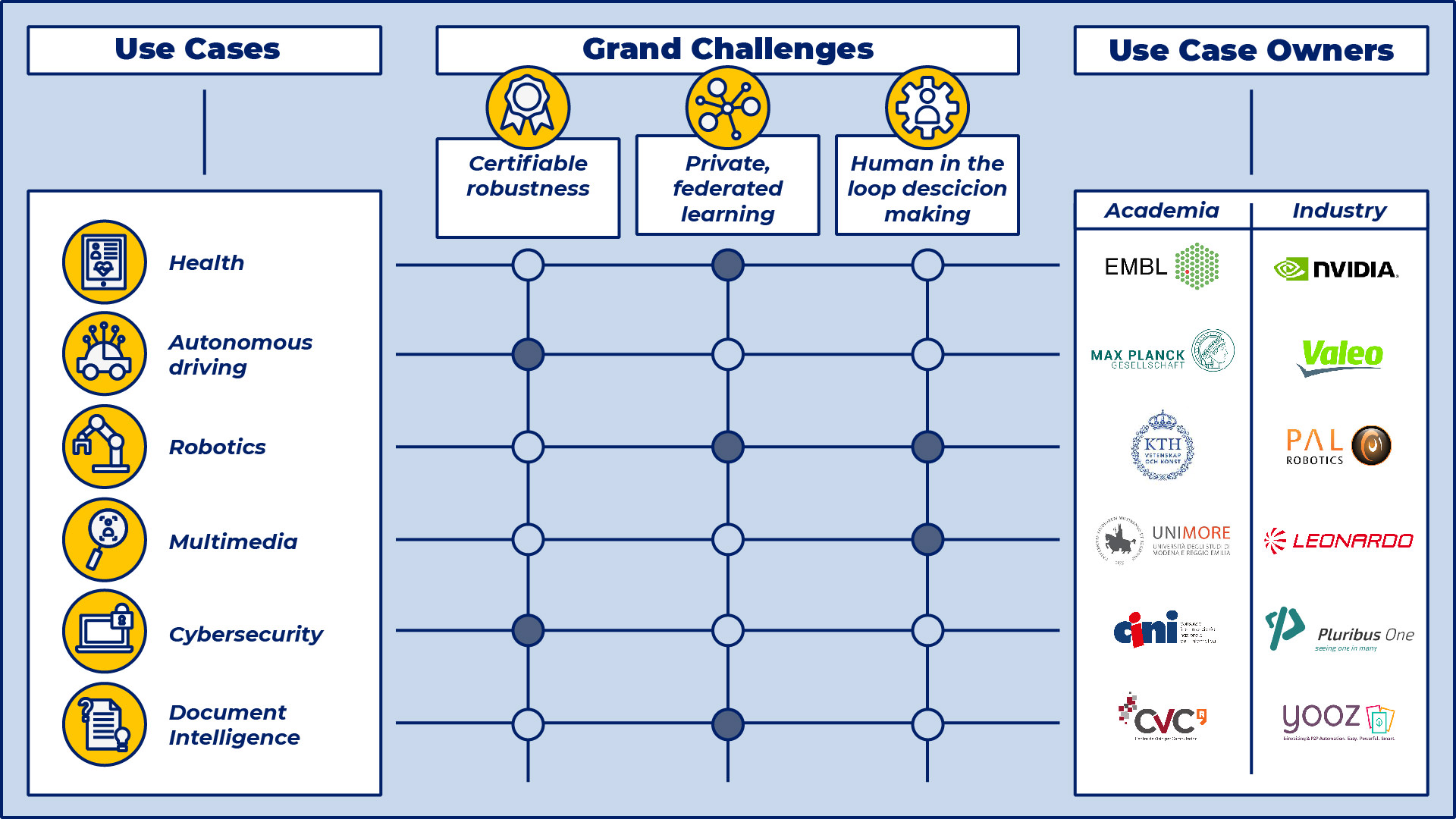
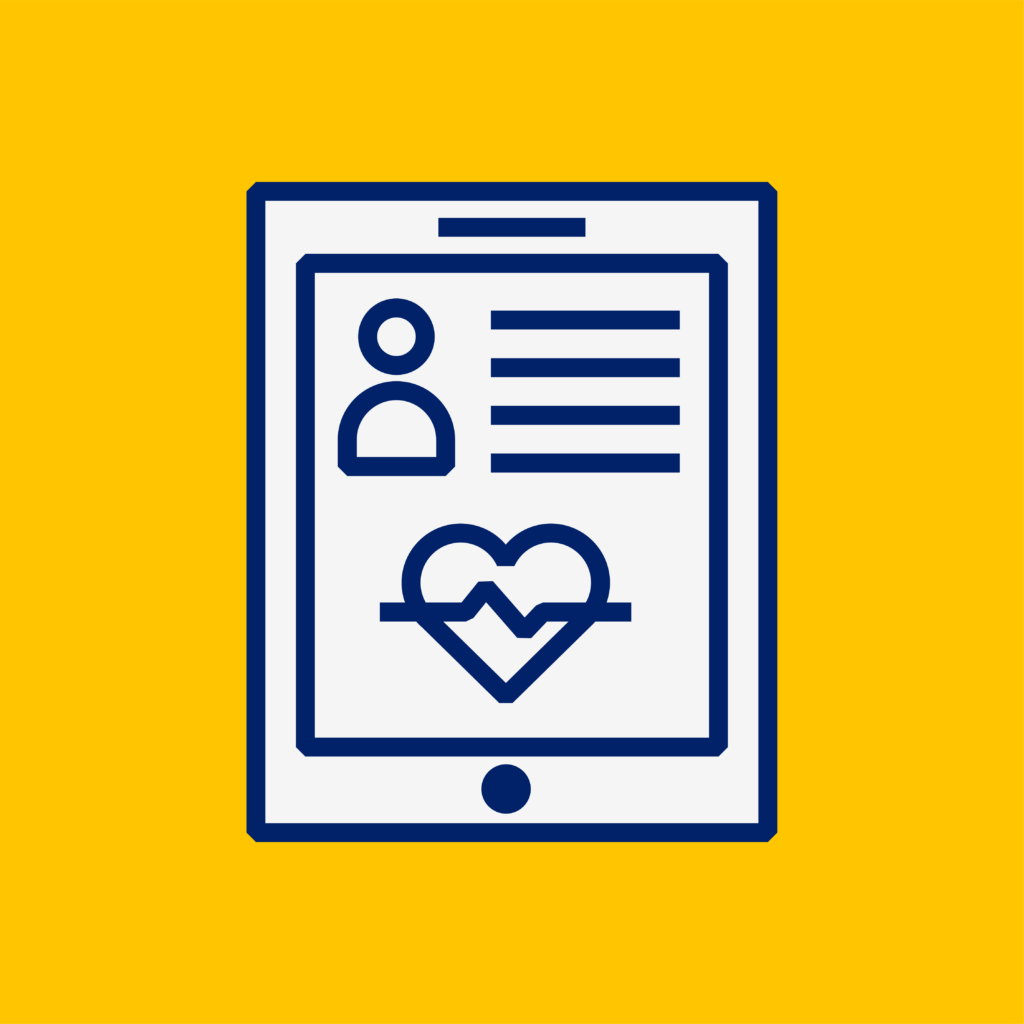
HEALTH – FEDERATED GENOME MEDICINE [EMBL, NVIDIA]
Health is sensitive and demands the highest standards of data and algorithmic safety, privacy and makes extensive use of federated inference and learning strategies. In this genome medicine use case, we will focus on genomic data and their integration with medical records and outcomes. This choice is motivated by emerging large-scale data resources and initiatives to integrate genome data and associated omics data modalities across Europe. Pertinent examples include the 1+ Million Genomes Initiative that seeks to connect genome data from European Citizens to deliver improved health care in rare disease, oncology and infectious disease. EMBL is operating the European Genome-Phenome Archive (EGA https://ega-archive.org), which is undergoing a transition towards a federated data model, which is serving as a key technology to underpin genome data sharing in Europe. At least five European countries are expected to join the federation model by 2022. This use case will deliver standards and establish proof of concept implementations to allow for federated data analytics for genome medicine.
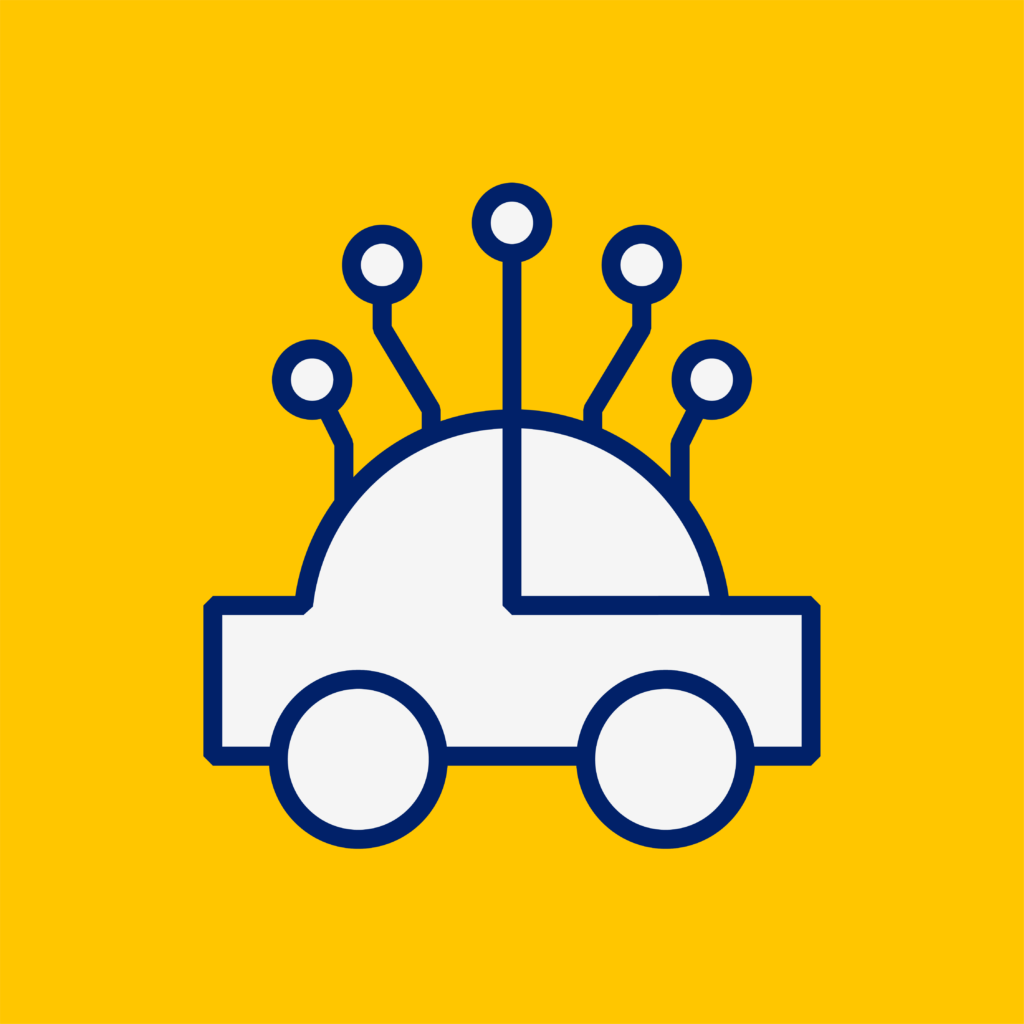
AUTONOMOUS DRIVING – ROBUST PERCEPTION [MPG, VALEO]
Autonomous vehicles are safety-critical systems operating in a widely complex open world; as such, they must not only deliver excellent performance in their operational design domain (ODD), but also be provably robust to all unexpected inputs caused by adversarial attacks, extreme weather conditions, changes of operation domains or rare but potentially catastrophic driving situations. We aim at developing testbeds to assess the robustness of driving perception models, so that it can be statistically proven. Relying on existing (possibly, augmented) or new datasets if suited, benchmarks with associated baselines will be set up to address the following safety-centered challenges: a) calibration of models’ outputs and estimation of their uncertainty; b) detection of out-of-domain inputs (either at scene or object level); c) assessment of gradual domain shifts away from the intended ODD.
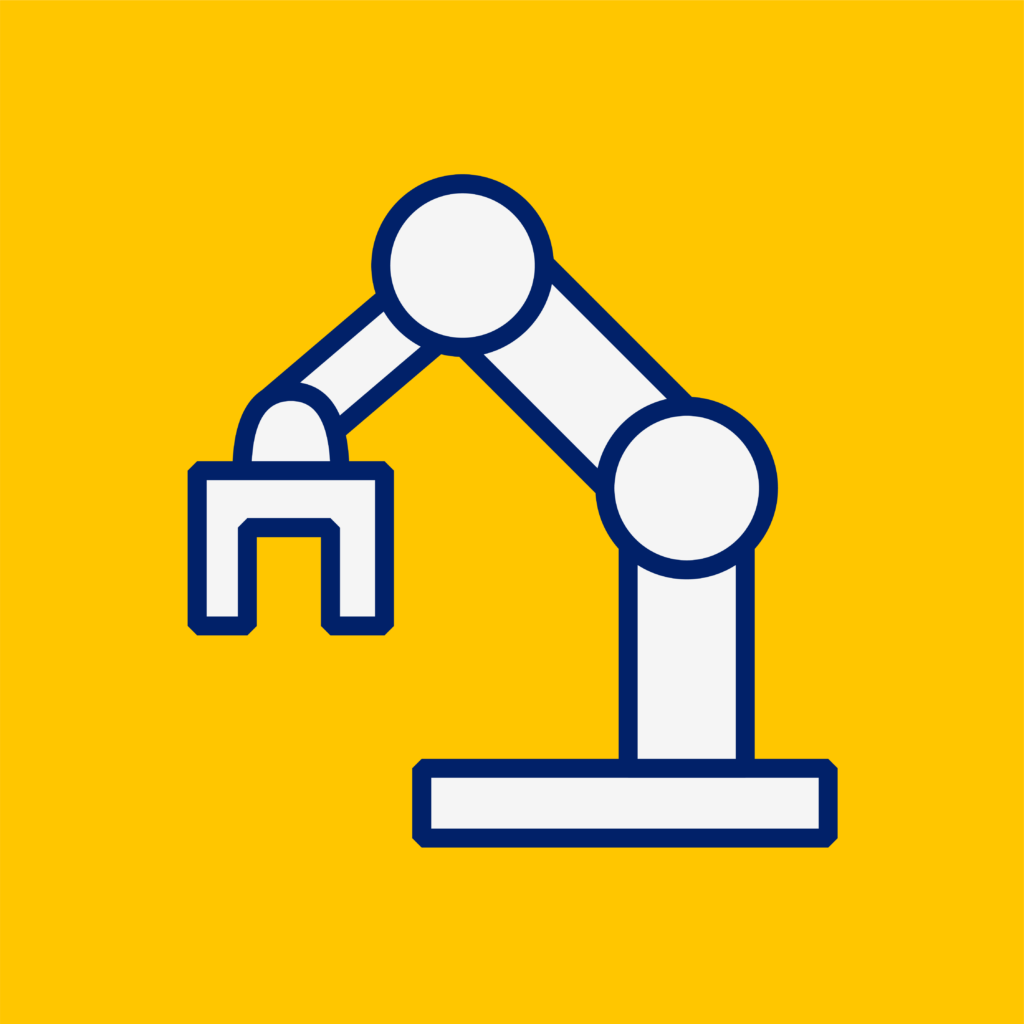
ROBOTICS – LEARNING THROUGH HUMAN INTERACTION [KTH, PAL]
Large scale deployment of robot systems in outdoor, industrial and domestic settings relies on safe and certifiable robustness; the ability to represent, abstract and transfer acquired knowledge through continuous learning; and doing this autonomously and/or with human-inthe-loop decision making. Human-in-the-loop enables efficient abstraction and transfer of knowledge, but it requires robust and reliable interaction that respects privacy. Training data based on human examples used in learning by demonstration scenarios need to ensure no private data are used or transferred. We will use and extend large-scale public datasets: a) AnDyDataset (https://andydataset.loria.fr), a dataset of industrial human activities to ensure that privacy is preserved; b) the KIT Whole-Body Human Motion Database (https://motion-database.humanoids.kit.edu), for learning whole-body manipulation tasks from multimodal human observation and their transfer to robots with different morphologies and include NLP interaction and c) RoboNet (https://github.com/SudeepDasari/RoboNet), an open database for sharing robotic experiences, to study privacy and federated learning.
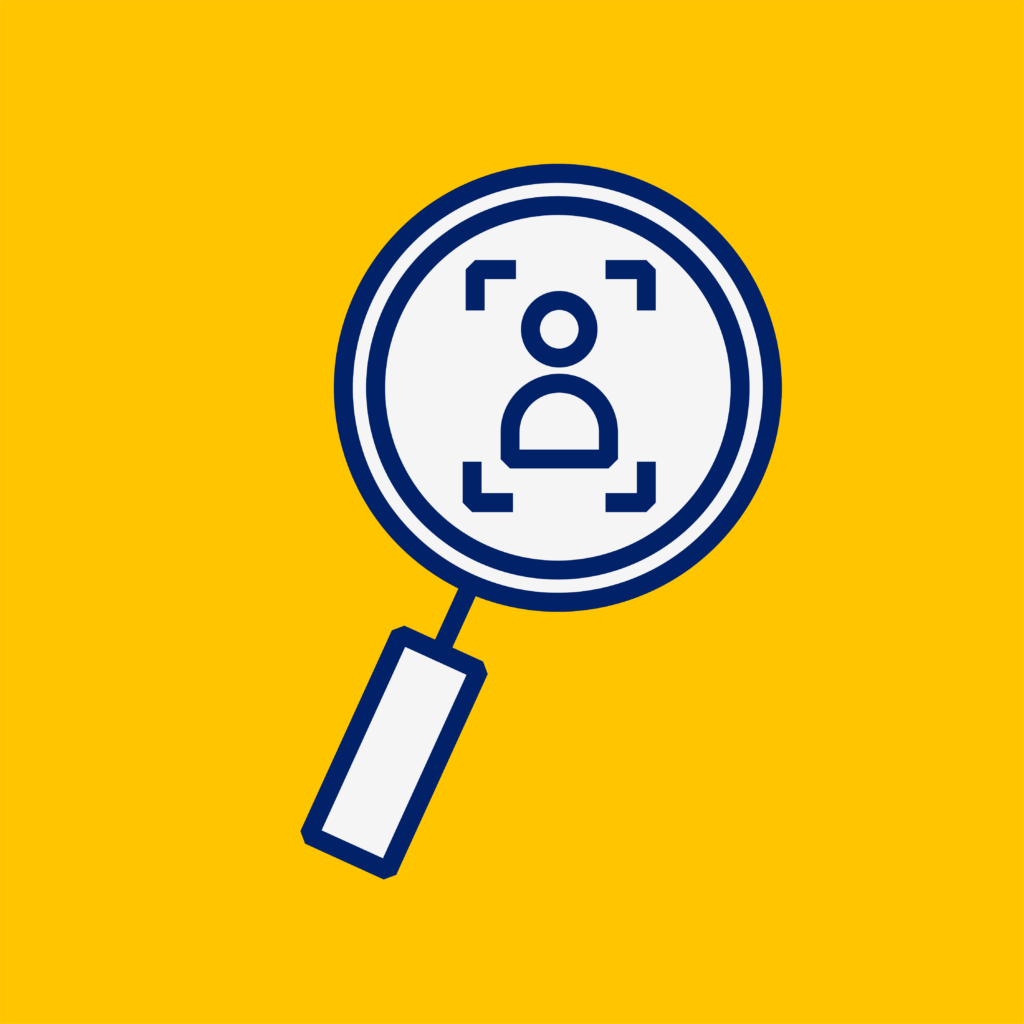
MULTIMEDIA – TACKLING DISINFORMATION [UNIMORE, LEONARDO]
Thanks to the power of deep learning techniques and novel architectures, images produced with generative networks are becoming increasingly realistic, enabling the creation of Deepfakes and helping to spread misinformation in media footage. While in the past few years generated images contained artefacts, today’s results are way less recognizable from a purely perceptive point of view. The use case will investigate novel ways of understanding and detecting fake data, through new machine learning approaches capable of mixing (a) syntactic/perceptive analysis, (b) semantic analysis and (c) human oversight analysis. While in the first scenario the approaches should work at a low level, evaluating the realism of pixels, shadows and textures, in the second scenario they shall evaluate the probability to have in a given context a given object, scene or action. This will also be carried out by mixing different media sources, e.g. by correlating visual and textual data. Finally, developed algorithms should benefit from human oversight by design, by enabling domain experts to validate and improve results in a human-in-the-loop fashion. The use case will be based on available initiatives to detect Deepfakes and misinformation scenarios, and will foster the creation of new datasets for misinformation detection.
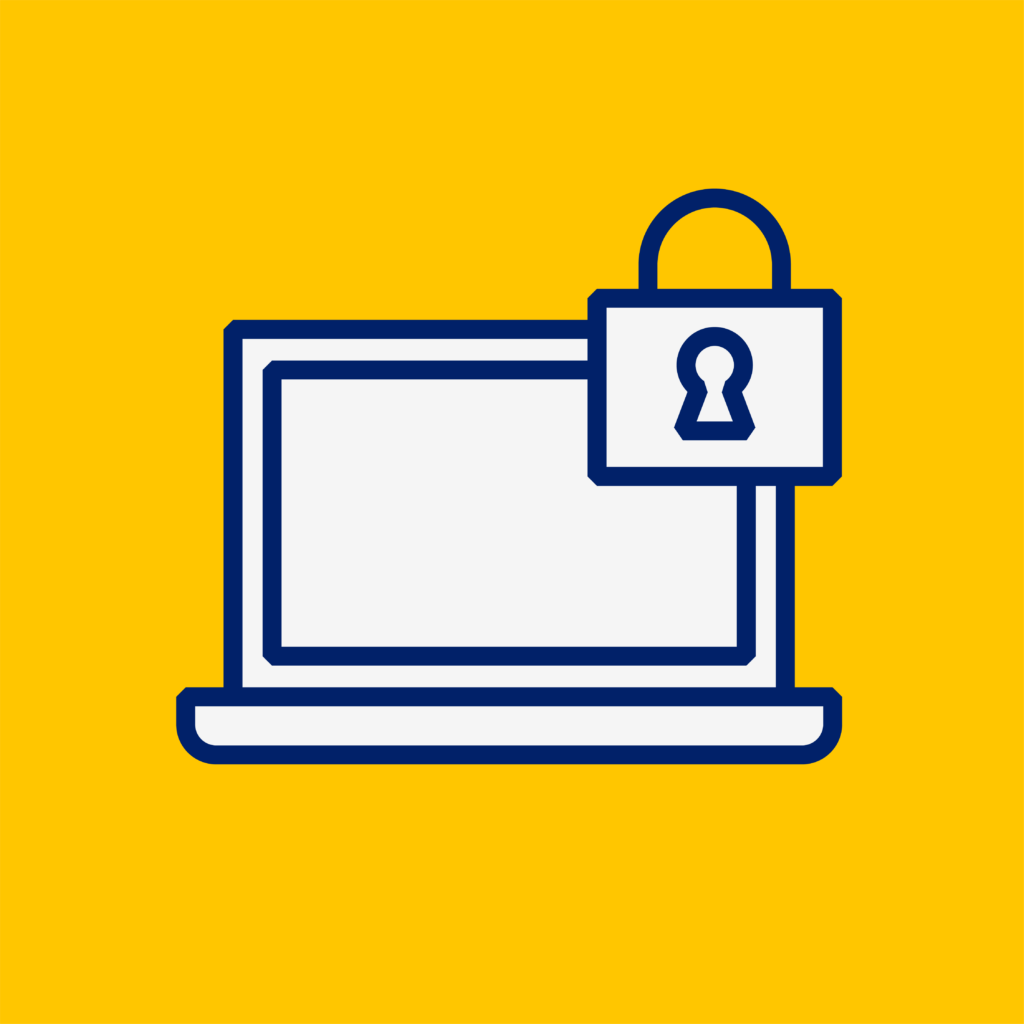
CYBERSECURITY – MALWARE DETECTION [CINI, PLURIBUS ONE]
Keeping end-user devices safe is a daunting and challenging task, as malware spreads across every kind of operating system. To cope with such an enormous amount of data, many anti-malware solutions are empowered by machine learning and data-driven AI algorithms. However, such algorithms fail to generalize well outside their training data distribution. The problem is exacerbated as (i) malware developers constantly manipulate their malicious samples to bypass detection and (ii) it is difficult to interpret the decisions of AI models and, consequently, their failure cases and how to mitigate them. To cope with these issues, AI models are frequently retrained on past and newly-collected data, demanding constant human intervention and dedicated resources. In this use case, we aim to overcome this problem by automating the process of building and deploying AI-based malware detection systems that can be maintained with less effort, and react more promptly to novel threats. We will base the use case on a continuous data collection via publicly-available sources (e.g., AndroZoo, VirusTotal, and VirusShare).
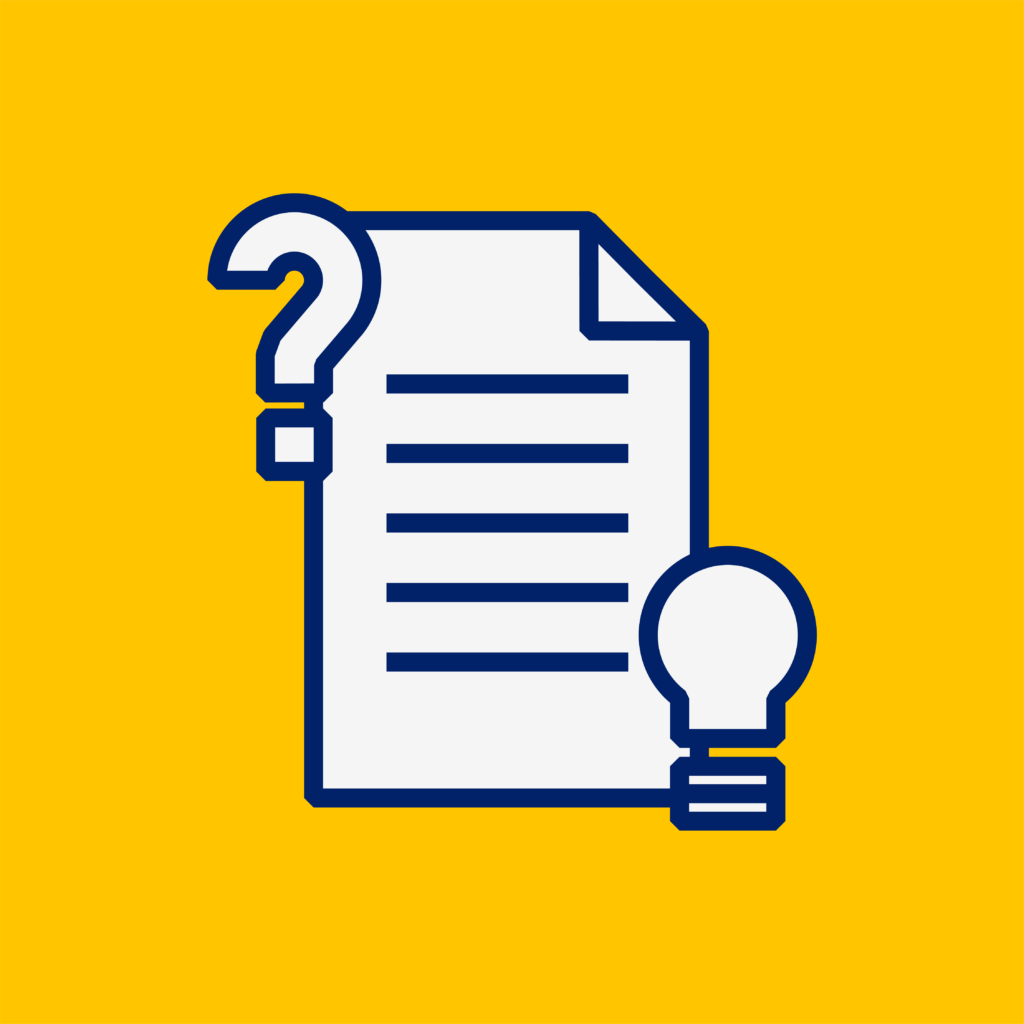
DOCUMENT INTELLIGENCE – DOCUMENT VQA [CVC-CERCA, YOOZ]
Automatically managing the information of document workflows is a core aspect of business intelligence and process automation. Reasoning over the information extracted from documents fuels subsequent decision-making processes that can directly affect humans, especially in sectors such as finance, legal or insurance. At the same time, documents tend to contain private information, restricting access to them during training. This use case seeks to explore methods for training large-scale models over private and widely distributed data. Specifically, we will explore novel techniques for training privacy-preserving document visual question answering systems over private data. We will base our benchmarks on repurposed large scale annotated datasets such as IIT-CDIP (Lewis et al., 2006), FUNSD (Jaume et al., 2019) and DocVQA (Mathew et al., 2021).